How aiApas Leverages Large Language Models (LLMs) for Enhanced Credit Risk Assessment in the Financial Sector
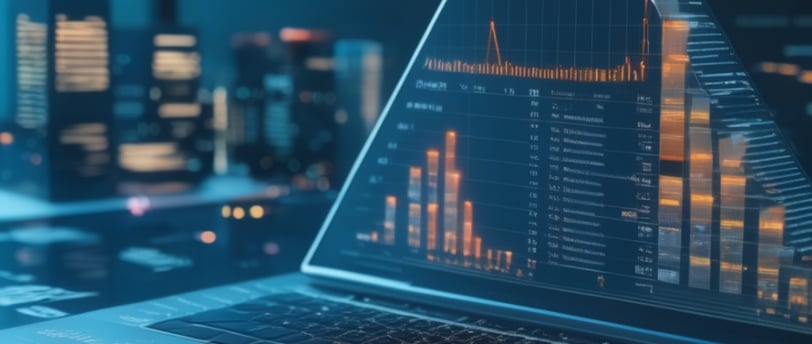
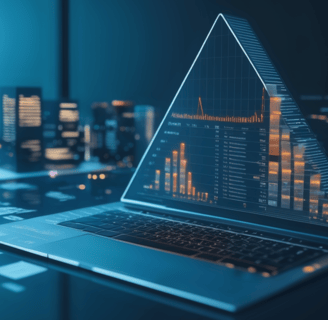
The Limitations of Traditional Credit Scoring Methods
Traditional methods of credit risk assessment, such as FICO scores, credit history, and debt-to-income ratios, have long been the cornerstone of banks and financial institutions. These metrics provide a standardized way to evaluate a borrower's creditworthiness, which has been instrumental in credit decision-making processes. However, these conventional models come with significant limitations that can lead to inaccurate or biased assessments.
One of the primary limitations is the reliance on historical data. FICO scores and similar metrics are heavily dependent on a borrower's past financial behavior, which may not always be indicative of their future performance. This backward-looking approach can overlook recent changes in a borrower's circumstances, such as a sudden job loss or a recent financial windfall, thereby failing to capture the complete picture of their current credit risk.
Moreover, traditional credit scoring methods often do not account for the complexities and nuances of borrower behavior. For instance, they may not consider non-traditional data sources like social media activity, rental payment history, or utility bills, which can provide valuable insights into a borrower's financial habits and stability. As a result, these methods can sometimes produce biased outcomes, disproportionately affecting individuals with limited credit histories or those from underserved communities.
The fast-paced and data-rich financial environment of today further exacerbates the limitations of traditional credit scoring methods. Financial institutions need to quickly and accurately assess credit risk to stay competitive and make informed lending decisions. However, traditional models are often slow to adapt to new data sources and analytical techniques, making them less effective in providing timely and precise risk assessments.
In this context, the limitations of traditional credit scoring methods underscore the need for more advanced and adaptable approaches to credit risk assessment. Leveraging modern technologies like large language models (LLMs) can offer a more nuanced and comprehensive understanding of borrower behavior, ultimately leading to more accurate and fair credit evaluations.
Large Language Models (LLMs) have emerged as transformative tools in the financial sector, particularly in the realm of credit risk assessment. Traditional credit scoring methods often rely on a narrow set of financial indicators, such as credit history, income, and debt levels. However, these indicators can be limited in scope and may not fully capture the complete financial behavior and risk profile of borrowers. aiApas leverages LLMs to overcome these limitations by harnessing their advanced capabilities in natural language understanding and generation.
LLMs, such as GPT-3 and similar models, are proficient in analyzing vast amounts of unstructured data, including social media posts, customer reviews, and financial news. This ability to process and interpret unstructured data enables LLMs to provide a more comprehensive view of a borrower's financial behavior and potential risk factors. By incorporating insights from diverse data sources, LLMs can identify patterns and trends that traditional methods might overlook.
For instance, social media activity can offer valuable clues about a borrower's lifestyle and spending habits, while customer reviews can reflect the stability and reputation of a business. Financial news can provide context on economic conditions that may impact a borrower's ability to repay loans. By integrating these diverse data streams, LLMs can deliver deeper insights into borrower behavior, allowing for more accurate risk predictions and personalized lending decisions.
aiApas has successfully implemented LLMs in credit risk assessment, leading to measurable improvements in decision-making processes. One case study involves a mid-sized lending institution that integrated LLMs to analyze customer interactions and financial news. The result was a 15% reduction in default rates and a 20% increase in loan approval speed. Another example is a fintech startup using LLMs to evaluate social media sentiment, resulting in more nuanced risk profiles and tailored lending products.
The practical benefits of LLMs extend beyond risk prediction to include enhanced customer experience. By providing more personalized lending decisions, financial institutions can better meet the unique needs of their clients, fostering stronger relationships and customer loyalty. The transformative impact of LLMs in credit risk assessment underscores their potential to revolutionize the financial sector, making lending processes more efficient, accurate, and customer-centric.